The next level of predictive maintenance
In cooperation with companies like WinGD and research partners like NASA, the ETH Intelligent Maintenance Systems Group is developing new methods for predicting machine failures. Manuel Arias Chao gives an insight into his project that he will present at this year’s Smart Maintenance Conference at ETH Zurich.
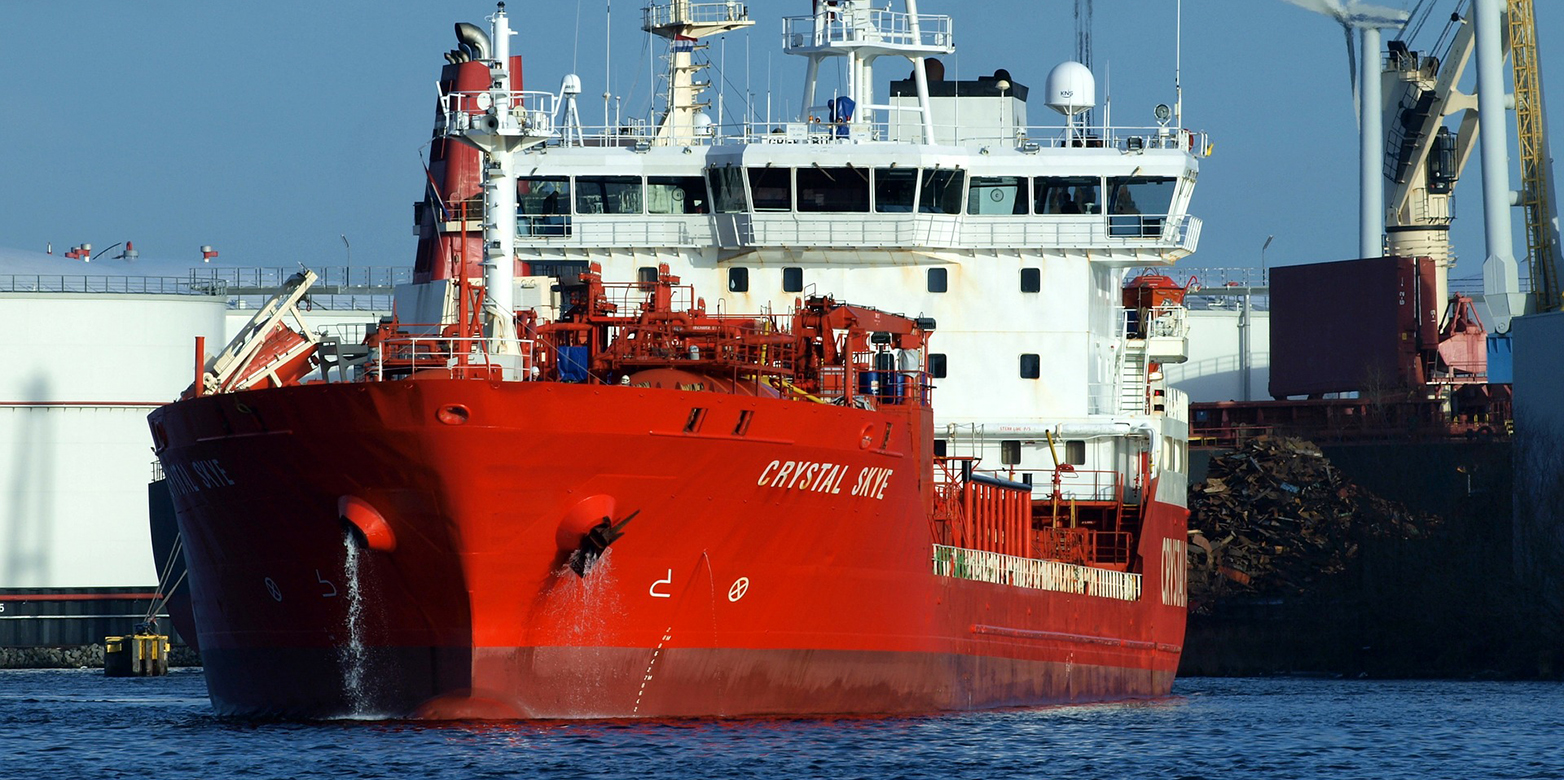
What was the motivation of WinGD (Winterthur Gas & Diesel) to initiate your project?
WinGD designs gas engines for large cargo tankers. Operating such complex systems requires dealing with many questions: When do the machines need maintenance? Will any component fail? Is the lifetime of a machine close to its end? Should we modify the operating regime of a machine in order to delay the time of failure?
Currently, WinGD is using a rule-based diagnosis and troubleshooting software. Rule-based means that engineers created rules defining how to deal with these issues. Now WinGD wants to digitalize their assets and implement remote monitoring of the machines. In this context, they want to apply machine learning in order to detect and locate failures. They started a joint project with Prof. Olga Fink who is a pioneer in the area of diagnosis and prognosis for complex systems. Personally, I am doing my PhD thesis in the scope of this project, focusing on a new hybrid approach.
What is special about your approach? Why do you call it hybrid?
Generally, the task is to recognize abnormalities in sensor data. Machines are usually equipped with many sensors, especially nowadays in the emerging IoT age. These sensors deliver data about process parameters (e.g. temperature, vibration, rotational speed). Degradation of components changes the machine behaviour. Algorithms can detect these changes and thus diagnose failures or – in a next step – also predict future failures.
There are two approaches for failure detection: either by using data-driven models or physical models. Data-driven models are based on condition monitoring data or experimental rules:
- Condition monitoring data: You build a numerical model from raw sensor data.
- Experimental rules: You carry out tests in the lab and get some correlations based on physical parameters.
Using physical models is very complicated, especially for large systems and can increase the development costs substantially. Therefore, they are typically only developed for expensive or critical equipment. The complex physical simulations require time-consuming calculations, and thus, are not suitable for all types of online monitoring.
Our new approach is to combine data-driven models with physical models (see image). We use physics as far as it is suitable for an online setting, e.g. we apply a thermodynamic model that only takes milliseconds to calculate. Apart from this hybrid aspect, we try to advance the data-driven part of the model further. Our new sophisticated data-driven models are based on deep learning and neural networks.
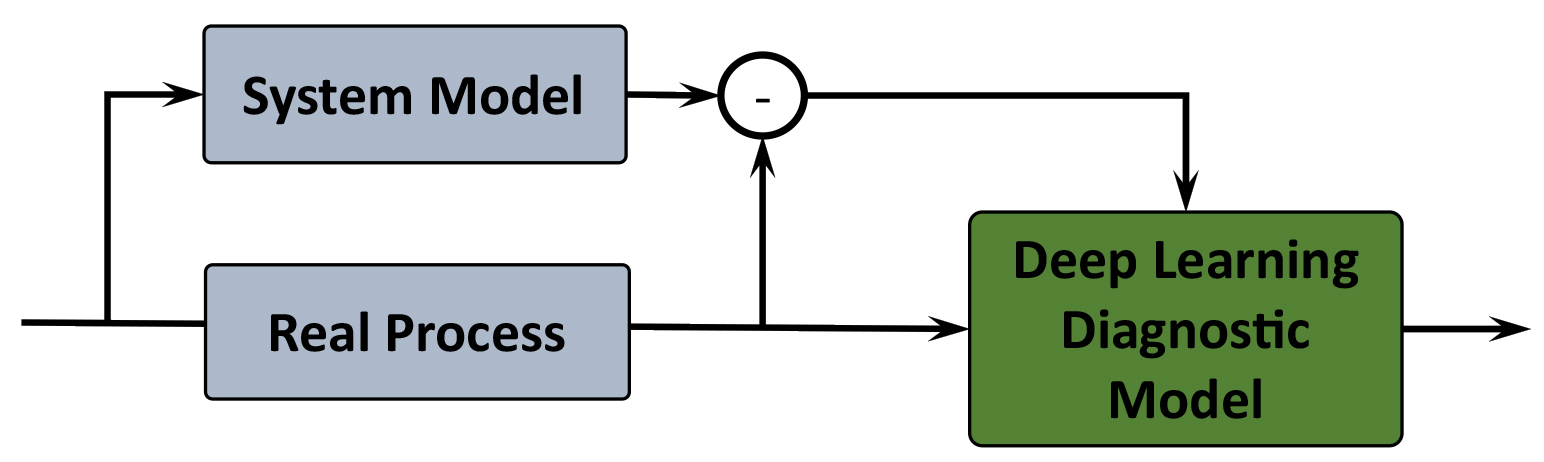
You are also collaborating with NASA on your research project. Why are they interested in your research?
NASA is particularly interested in our hybrid approach and its application to turbofan engines. We defined a nine months collaboration with the Prognostics Center of Excellence (PCoE) at NASA Ames. I stayed in Mountain View for the first three months of 2019. During my stay, we focused on the development of a new data set to test the method and to further develop the hybrid framework.
Have you tested your algorithms with your partners?
We tested our algorithms with WinGD as well as with NASA’s data sets. These tests showed that we are more accurate on detecting failures than standard approaches. Furthermore, we can differentiate between different types of failures (see image). WinGD plans to implement our solution in the next version of their diagnosis and troubleshooting software.
Currently, we are extending our hybrid approach from diagnosis to prognosis. That’s what I will present at the Smart Maintenance Conference at ETH Zurich on 3rd September.
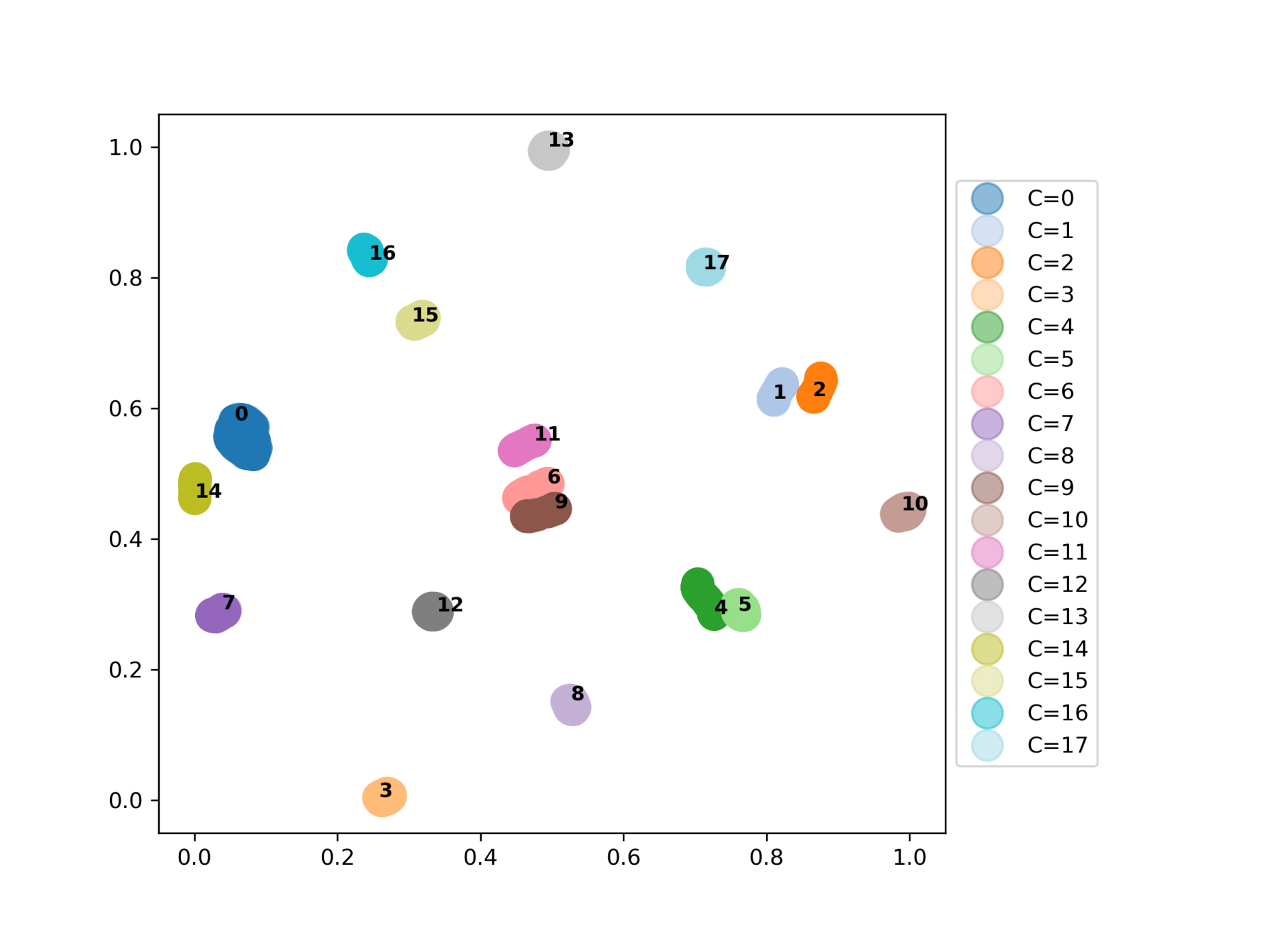
Tell us more about the Smart Maintenance Conference.
Prof. Olga Fink organises this conference for the third time. Our aim is to foster the exchange between industry and academia. The talks are given by representatives of well-known companies as well as academic experts. It is always great to meet the key academic and industrial players in predictive maintenance to discuss the challenges and new developments in the field.
Contact / Links:
external pageSmart Maintenance Conference 2019call_made
Prof. Olga Fink, ETH Intelligent Maintenance Systems Group
Do you want to get more "News for Industry" stories?
external pageSubscribe to our newslettercall_made
external pageFollow us on LinkedIncall_made
Are you looking for research partners at ETH Zurich?
Contact ETH Industry Relations