Our actual attention is now measurable
We want to make sure our phones no longer disturb us at the wrong moment. To achieve this, we first have to better understand where our attention lies when using smartphones. Computer scientists at ETH have now developed a system that records eye contact with the display in everyday situations for the first time. Sociologists and medical experts could also benefit from this.
How many times a day do you turn on your smartphone? How long is the screen on and how long are the various apps in use? Every modern smartphone collects this data automatically and makes it available to the user under headings like “Digital wellbeing”. But not all screen time and app use is equal. Sometimes we concentrate fully on something for a long time, while at other times we only look briefly at the screen or are distracted multiple times by things going on around us. And sometimes we don’t look at our smartphone at all, because we’ve activated it by accident.
The key to attentive user interfaces
“The level of attention we pay to our smartphones can vary considerably,” explains Mihai Bâce, “but this has never been examined in real-life everyday situations.” Together with a Master’s student and a professor from the University of Stuttgart, Bâce, who is a doctoral student at ETH Zurich’s Institute for Intelligent Interactive Systems, has developed a system to measure the visual attention paid to a smartphone during a user’s normal day over the course of weeks. All it requires is the front-facing camera and the phone’s sensor data. Previously, researchers had to use cumbersome measuring apparatus with eye trackers or ask participants to fill out surveys, which could at best only approximate normal life.
Understanding user attention is one of the most important challenges on the path to future mobile user interfaces, emphasises Bâce. These should be attentive and automatically take into account our current needs and the situation we are in. Then, for example, there will no longer be any need for a manual “do not disturb” setting to avoid being torn away from a concentrated activity by an unimportant notification.
Only 7 seconds at a time and distracted 4 times
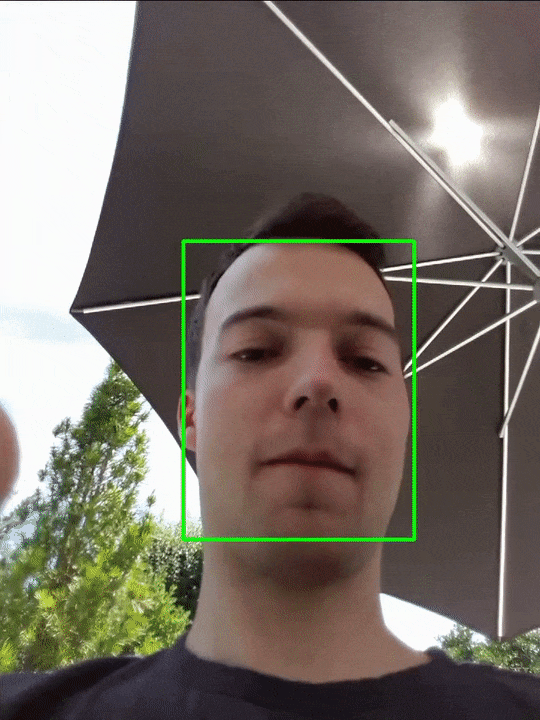
This kind of technology seems to be becoming ever more necessary: Bâce’s research shows that the visual attention we give to smartphones is currently extremely fragmented.
On average, eye contact with the screen lasts only seven seconds before the gaze wanders – and this happens four times every time the phone is unlocked, for about two seconds each time.
The user’s level of distraction depends on their individual personality, but also on their environment and the type of app currently in use. For example, medical apps or those used for training or education keep people’s attention better than entertainment apps.
Basis for research in a wide range of areas
For Bâce, however, the major value of his work does not only lie in the concrete research results that can be obtained using the system: “Above all, we want our system to provide a basis for other scientists. We will therefore publish all our algorithms in addition to all the video data.”
App developers are not the only ones who could benefit in future: sociologists or psychologists could also use the system to carry out studies on the influence of various factors on attention without any great technical outlay. The medical field could also make use of the technology: for example, changes in attention behaviour could be checked when monitoring patients, and could point towards problematic developments.
When developing the system, an app was used that, in addition to recording videos using the front-facing camera each time the phone was unlocked and collecting various sensor and metadata in parallel, also contained data protection and verification features.
The study participants were able to use a review component to decide for themselves which videos to release for evaluation, and video sequences could be evaluated by other participants via an annotation game. The results of the automatic eye contact detection were reviewed during the development phase with the help of this third component.
Infrastructure was a great challenge
In an initial experiment with 32 participants and over a period of more than two weeks, the researchers recorded video sequences totalling 472 hours and then evaluated them using an innovative adaptive eye contact detection system. The individual videos could be up to several hundred megabytes in size, which meant that a lot of storage space was required on the smartphones and the upload times were correspondingly long. This was one of the greatest challenges.
Since users quickly switch off or at least minimise the use of apps that interfere with their everyday life, mechanisms had to be found to avoid placing an excessive load on the smartphone’s memory or blocking its transmission capacities.
Data protection also had to be ensured at all times – only content that had been explicitly released by the users using the review component could be uploaded to the evaluation server. “The app was checked by ETH Zurich’s Ethics Commission, and we also are deliberately not conducting any facial recognition. We’re only examining if there is eye contact with the screen,” explains Bâce.
Our smartphones will not necessarily have to evaluate sensitive personal data in order to understand us and our needs better in future. Instead, the computer scientists’ system could help to achieve this through the automatic detection of people’s attention levels.
References
Bâce M, Staal S, Bulling A. (2020). Quantification of Users’ Visual Attention During Everyday Mobile Device Interactions. In: Proceedings of the Conference on Human Factors in Computing Systems (CHI ’20). ACM, New York, NY, USA, 2020, 1–14. doi: external page10.1145/3313831.3376449call_made
Bâce M, Staal S, Bulling A. (2019). Accurate and Robust Eye Contact Detection During Everyday Mobile Device Interactions external pagearxiv.org/abs/1907.11115call_made
Video presentation of the paper: external pagewww.youtube.com/watch?v=SzLn3LujIqwcall_made
Video dataset from the study: external pagewww.emva-dataset.orgcall_made