Forum on "AI in STEM Education"
The ETH AI Center is hosting the "AI in STEM Education" forum, discussing various applications and perspectives of AI in science, technology, engineering, and mathematics higher education.
Date: 16 May 2024
Time: 15.00 – 19.00 (including apéro riche and networking event)
Location: Zürich Oerlikon OAT X 11 ETH AI Center, Andreasstrasse 5, 8092 Zürich
Language: English
Registration is limited and required: external page https://forms.gle/bD6iC9nm6F4yASzg9
Using ChatGPT4 in physics education
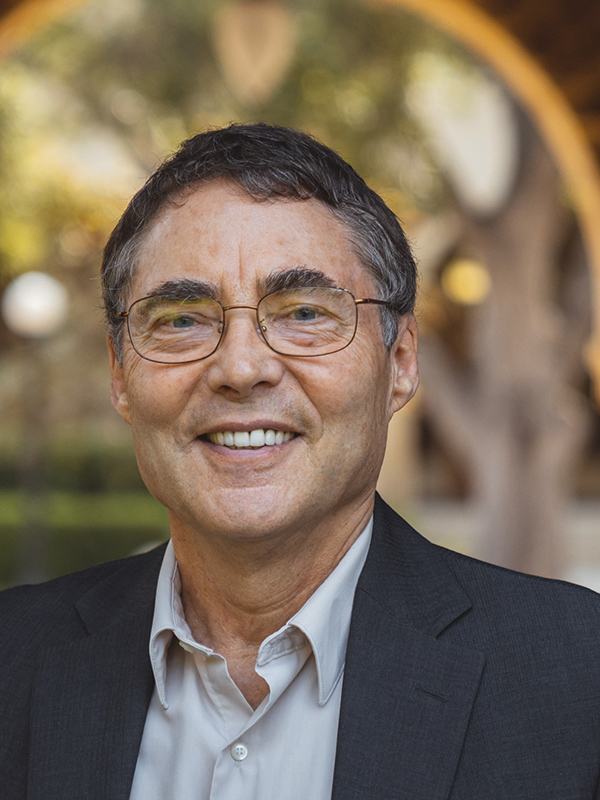
Carl Wieman, Stanford University
My group has been investigating the opportunities and challenges of using ChatGPT4 in a variety of ways in teaching introductory physics. This includes evaluating its strengths and weaknesses at: solving different types of homework problems; evaluating student solutions; and providing tutor-like feedback to students. We have had the most success with using it to grade and provide feedback on student work associated with doing well-structured assessment tasks.
Carl Wieman is an Emeritus Professor of Physics and Education at Stanford University. Wieman has been widely recognized for his experimental research in both atomic physics (Nobel Prize 2001 and other awards) and university science and engineering education (Carnegie University Professor of the Year 2004, Yidan International Prize for Education Research 2020). Wieman directed the Science Education Initiatives at the Universities of Colorado and British Columbia which were produced large scale change in the teaching science. He also served as Associate Director of Science in the White House Office of Science and Technology Policy from 2010 to 2012. He founded PhET, which provides interactive simulations that are used nearly a million times a day to learn science, and he has published a book "Improving how universities teach science." He is studying problem solving expertise in science and engineering and how this can be better measured and taught.
From Prediction to Generation: AI in Physics Education
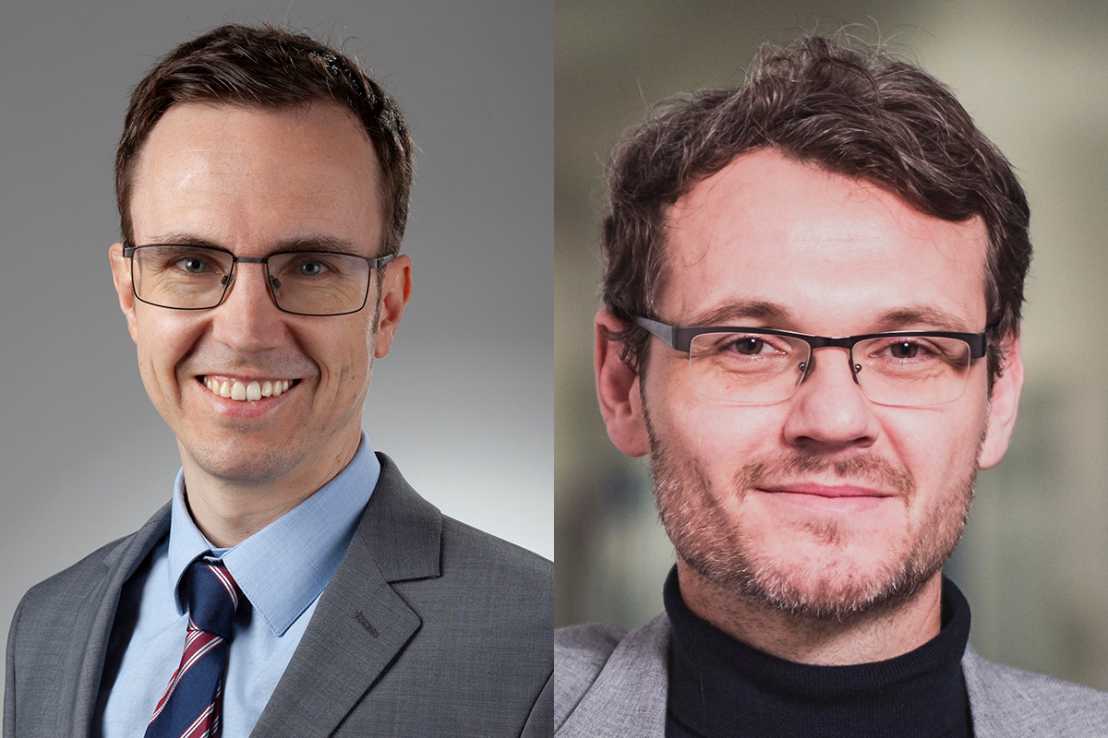
Stefan Küchemann and Jochen Kuhn, LMU Munich
Until the end of 2022, AI had played only a minor role in physics education, if at all, mostly with the focus on the possibilities of AI in the evaluation of extensive or heterogeneous data sets, as they arise in learning with digital technologies. With the public availability of ChatGPT since the end of 2022, generative and other AI tools have come more in the focus of physics education research. In this talk, we highlight these broad aspects showing possibilities and challenges of AI-based assistance in different learning scenarios. Specifically, we provide an overview of various roles of AI in learning environments to support conceptual understanding, self-regulated learning and critical thinking. In this way, we demonstrate how common challenges, such as hallucinations, misuse, and the need for prompting strategies can be avoided.
Jochen Kuhn holds the Chair of Physics Education Research in the Faculty of Physics at LMU Munich. His research focuses on learning and problem solving with multiple representations using future technologies as well as teaching and learning with and about artificial intelligence in STEM subjects. He installed the Immersive Quantified Learning Lab (iQL) at the German Research Center for Artificial Intelligence and is elected Fellow of the Konrad Zuse School of Excellence in Reliable AI (relAI). There he coordinates the research area “AI in Education”.
Stefan Küchemann is the head of the Junior Research Group on AI in Physics Education at the Chair of Physics Education Research. His research focuses on the development of AI tools for STEM education and on research regarding their effective integration in learning environments. Furthermore, he uses eye tracking to obtain insights into students’ learning and problem solving processes to provide real-time learning support.
Could ChatGPT be an Engineer? Evaluating Higher Education Vulnerability to AI Assistants
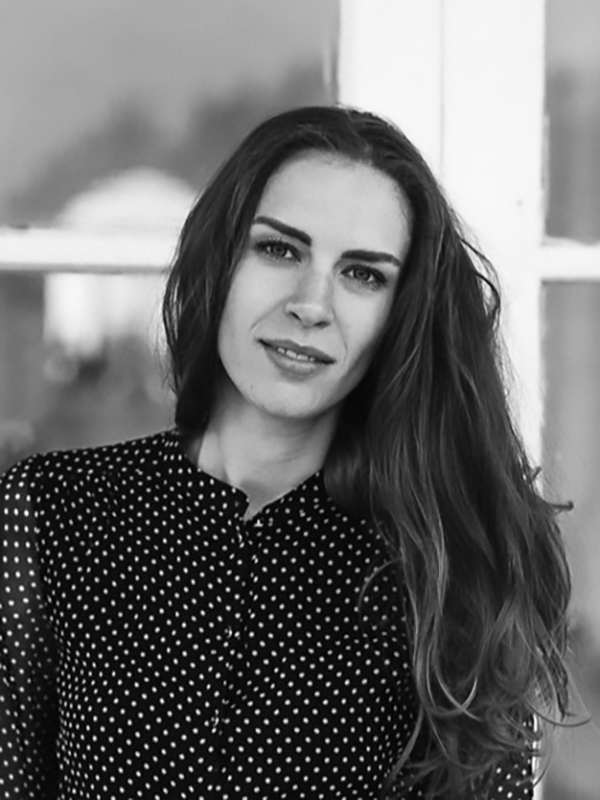
Anna Sotnikova, EPFL
The rapid adoption of generative AI technologies like large language models (LLMs) in educational environments poses significant challenges as students increasingly integrate such tools into their academic routines. Worryingly, these tools could be used in assessment settings, where their capabilities would provide an unfair advantage to their users and obfuscate downstream measures of student performance that universities are publicly relied upon to provide. In this work, we measure the risks of this vulnerability and investigate whether LLMs can successfully complete assessments in standard university-level STEM courses. Specifically, we compiled a novel dataset of assessment questions from 55 STEM courses at EPFL and evaluated whether GPT-3.5, a freely available online system, and GPT-4, the top-performing LLM agent (available through paid subscription), can successfully answer these questions. Using eight prompting strategies to produce responses, we find that the GPT-4 model achieves answers an average of 65.6 % of questions correctly in each course (when selecting responses by majority vote across prompting strategies) and that the model can produce the correct answer across at least one prompting strategy for 84.5 % of questions. When grouping these courses by program, we find that large numbers of core courses in various university degrees are already successfully passed by these models, posing significant future risks to higher education assessment and accreditation, especially as these models continue to improve. Finally, we discuss potential changes to university evaluation to mitigate these vulnerabilities and strengthen university evaluations.
Anna Sotnikova received her Ph.D. in Applied Mathematics at the University of Maryland under the guidance of Prof. Hal Daumé. Her research focuses on the intersection of Natural Language Processing (NLP) and ethics, with a particular emphasis on biases, transparency, and accountability in artificial intelligence. She is currently a postdoctoral researcher at EPFL Computer Science Department, where she works in the NLP lab under the supervision of Prof. Antoine Bosselut.
Towards a Literate AI and AI Powered Intelligent Learning Tutoring Systems
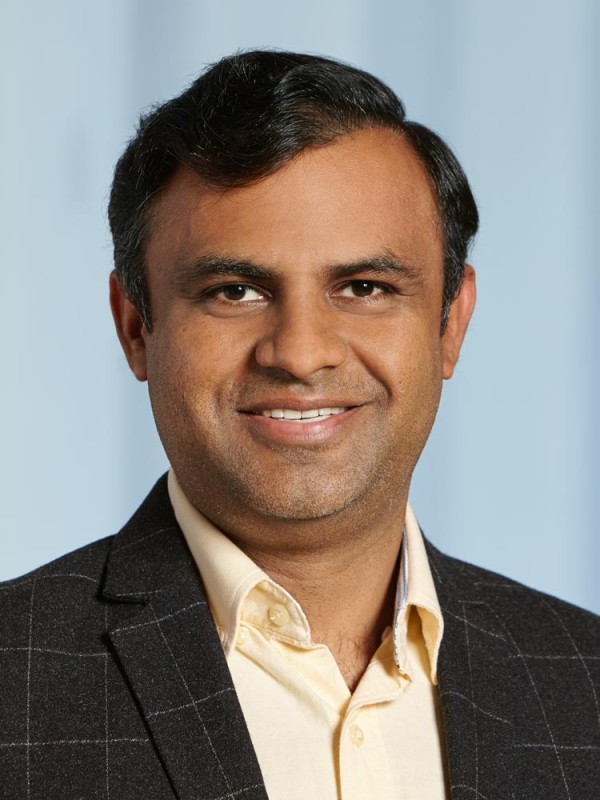
Mrinmaya Sachan, ETHZ
I will present some of our recent work on leveraging large language models (LLMs) to support education in various ways, such as creating practice problems and scaffolds, providing meaningful feedback to students, having a Socratic dialog with students, etc. - while - customizing the learning experiences of students according to their own individual needs. Finally, I will share some challenges as well as opportunities in this emerging field.
Mrinmaya Sachan is an Assistant Professor of Computer Science at ETH Zurich. His research is in the area of Natural language processing and the interface of Machine learning and Education. Prior to this position, Mrinmaya was a Research Assistant Professor at TTI Chicago. Before that, he received a Ph.D. from the Machine Learning Department at CMU and a B.Tech. in Computer Science from IIT Kanpur where he received an Academic Excellence Award. He has received several awards for his work, including an outstanding paper award at ACL and EMNLP, an IBM PhD fellowship, the Siebel scholarship and the CMU CMLH fellowship. His current research is funded by grants from the Swiss National Science Foundation, the ETH Zurich foundation and Haslerstiftung.
Ethel - a virtual teaching assistant
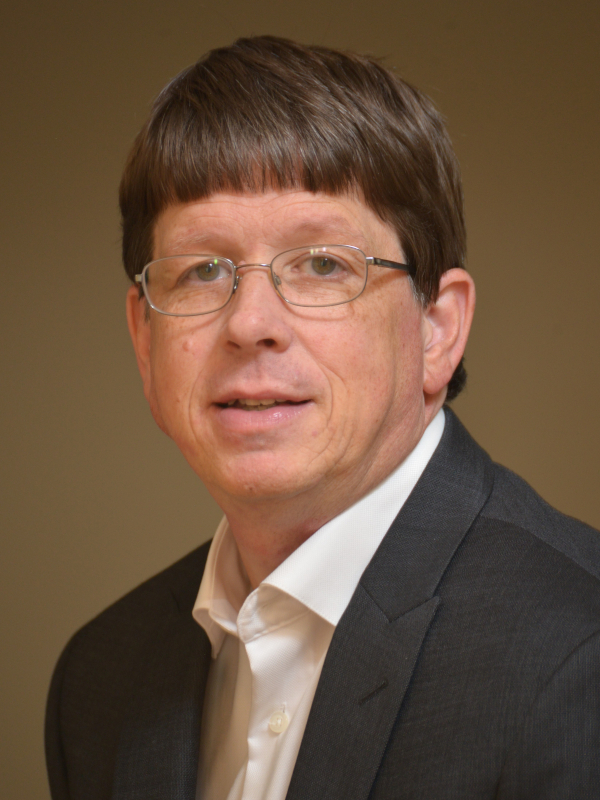
Gerd Kortemeyer, ETHZ
Can an AI be an additional teaching assistant for a STEM course? Can it help with assignments, give formative feedback on solutions, support students when preparing for exams, and reliably support the grading of exams? The talk describes Project Ethel, an effort to provide a course-specific virtual teaching assistant, and it relates experiences gathered with the system across various courses and tasks. It also provides an outlook on future plans, pointing out challenges and opportunities for supporting learning in STEM disciplines using AI.
Gerd Kortemeyer is a member of the rectorate and the AI Center of ETH Zurich, where he advances initiatives in the area of “AI for Education." He holds a Ph.D. in physics from Michigan State University, where he is also an Associate Professor Emeritus of Physics Education. For decades he has been active in research and development of educational technology, particularly in the areas of formative assessment and learning analytics.