Artificial intelligence accelerates blood flow MRI
Imaging technology helps to detect cardiovascular diseases much earlier; however, precise examinations are still very time-consuming. Researchers from ETH and the University of Zurich have now presented a method that could greatly accelerate dynamic magnetic resonance imaging of blood flow.
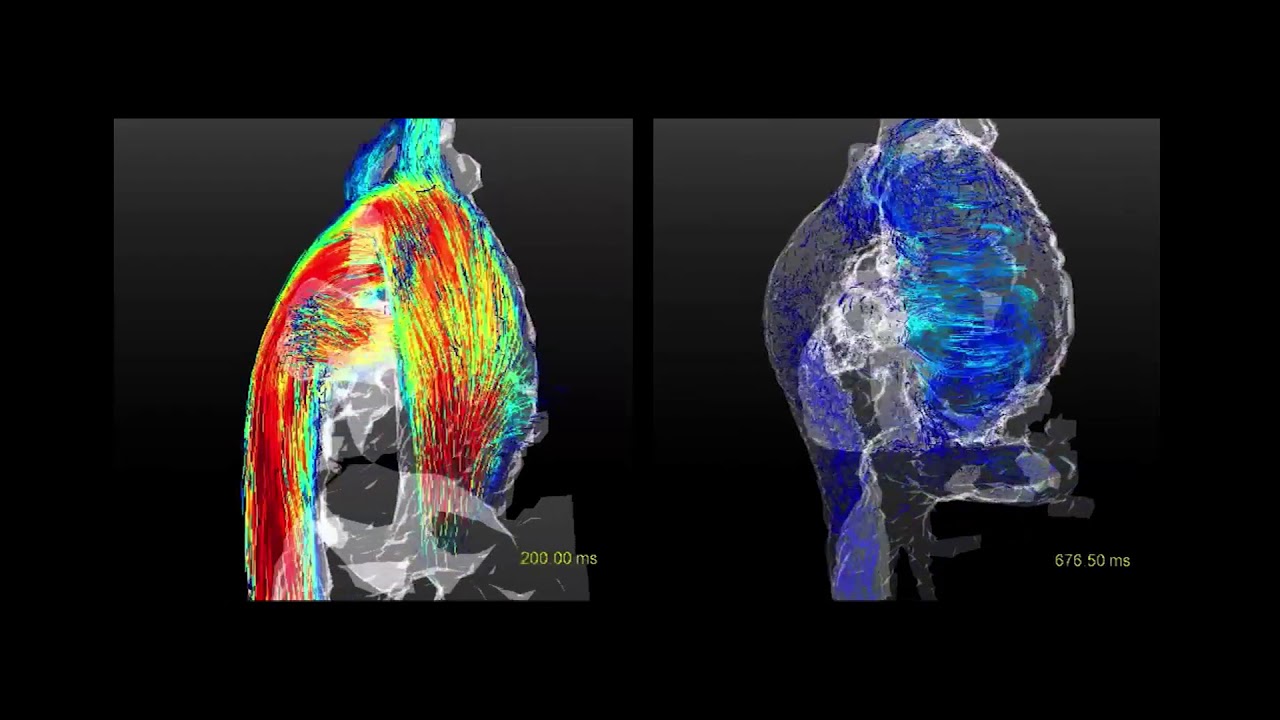
“Thanks to this innovation, quantitative magnetic resonance imaging could make tremendous progress,” says Sebastian Kozerke, Professor of Biomedical Imaging at ETH and the University of Zurich. He worked with Valery Vishnevskiy and Jonas Walheim to develop a method that greatly accelerates so-called 4D flow MRIs.
“At the moment, the recording and subsequent processing of a 4D flow MRI takes up to 30 minutes. Our results show that this could be possible within five minutes in the future.” The underlying research was featured in the journal Nature Machine Intelligence earlier this week as article and cover of the April issue.
Magnetic resonance tomography (MRT or MRI) is a key modality in clinical diagnosis. It poses no health risks and provides precise images of the interior of the body. This method can be used to display soft body parts such as tissue and organs in 3D and with high contrast. Furthermore, special recording techniques deliver information on the dynamics of the cardiovascular system.
In particular, 4D flow MRI measurements enable the quantification of dynamic changes of blood flow. Such dynamic images are highly useful, particularly when it comes to detecting cardiovascular diseases.
However, conventional 4D flow MRI has a significant drawback: the method is very time-consuming. Nowadays, the data recording can be completed in the MRI scanner within four minutes. However, the required compressed sensing approach comes at a cost: the subsequent image reconstruction is iterative and thus takes a very long time. Doctors have to wait 25 minutes or longer for the images to appear on their computers.
Thus, the results of the measurement only become available long after the doctor has completed the examination. This is why 4D flow MRI is not yet established in everyday medical practice. Changes to blood flow are currently diagnosed primarily via ultrasound – a method that’s quicker but less precise in comparison with MRI.
Elegant and efficient algorithms
In the recently published article, the researchers from ETH and the University of Zurich illustrate a way in which image reconstruction for 4D flow MRI could be made quicker and thus more practical. “The solution consists of elegant and efficient algorithms based on neural networks,” explains Kozerke.
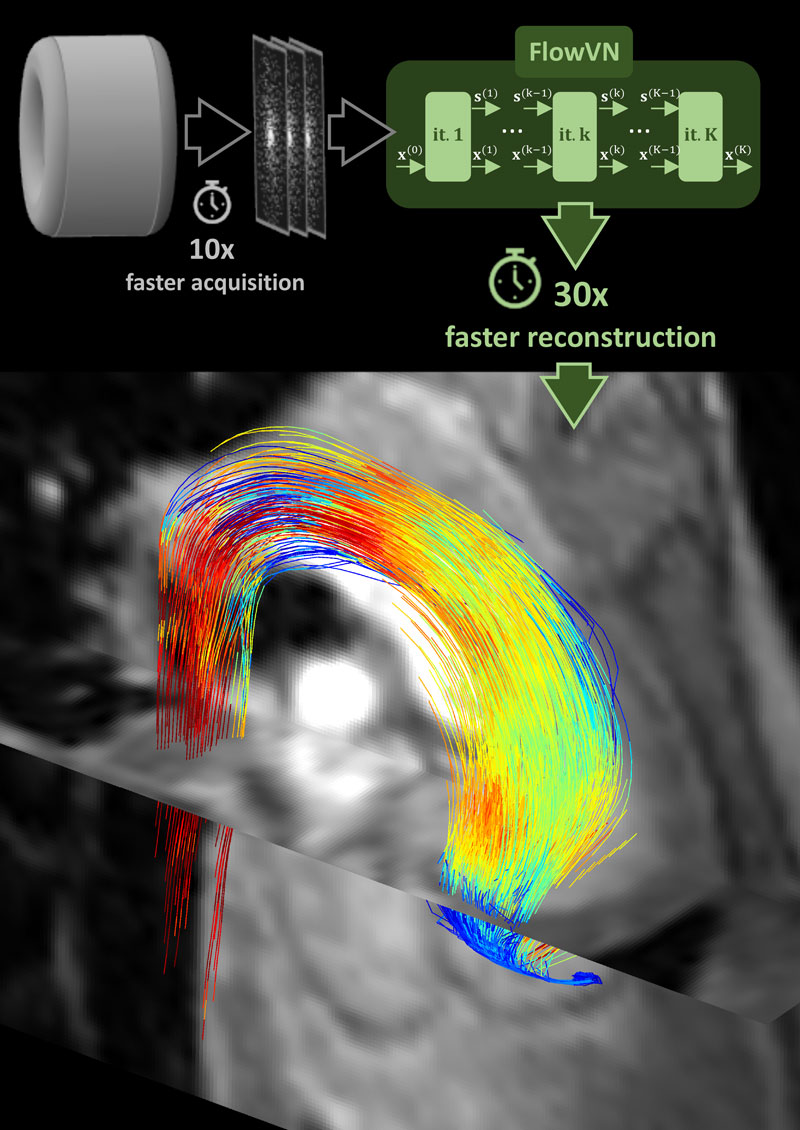
Vishnevskiy, Kozerke and Walheim call their new approach FlowVN. It is based on machine learning, more specifically on what is known as deep learning; the software learns through data presented during a training stage. What makes FlowVN so special is the efficiency – the method combines training with prior knowledge of the measurement.
This means that generalisations can be made on the basis of little data instead of requiring thousands of training examples. “As a result, the network needs very little training to deliver reliable results,” explains Vishnevskiy.
The researchers were able to demonstrate that this method works as described in thier recently published paper. They trained the software using 11 MRI scans of healthy test subjects. This data was sufficient to accurately reproduce pathological blood flow in a patient’s aorta on an ordinary computer within just 21 seconds. The method is thus many times faster than conventional methods – and, on top, delivers better results.
Advancing clinical diagnosis
“We hope that FlowVN will drive forward the use of 4D flow MRI in clinical diagnostics,” says Kozerke. The data was reconstructed offline for this study. The next step for the Zurich research team will be to install the software on clinical MRI machines. “We then envisage larger clinical patient studies,” says Kozerke. The researchers benefit from the long-term partnership with the radiology and cardiology departments at the University Hospital Zurich.
If the follow-up tests confirm the results obtained by Kozerke’s team, the method could one day make its way into everyday medical practice. “However, it will take at least another four or five years until this happens,” estimates Kozerke. In order to accelerate the scientific research process, his team made the executable codes and data examples available as open source, enabling other scientists to test and reproduce the method.
Reference
Vishnevskiy V, Walheim J, Kozerke S. Deep variational network for rapid 4D flow MRI reconstruction. Nature Machine Intelligence, 13. April 2020. doi: external page 10.1038/s42256-020-0165-6