Rainforest preservation through machine learning
Computer scientist David Dao develops intelligent algorithms that use satellite and drone images of rainforests to predict where the next sites of deforestation will be. He will be presenting his research at the climate conference in Madrid today, and will start a pilot project in Chile in January.
Images of burning rainforests in the Amazon region were seared into our memories this summer and raised the question – how much of the forest have we lost? One of the people attempting to answer this question is computer scientist David Dao, a doctoral student from the external pageDS3Labcall_made at the ETH Institute for Computing Platforms.
Dao, who is originally from Germany, is a specialist in machine learning and develops intelligent algorithms that can autonomously analyse satellite and drone images. This helps to reveal where forest coverage is thinning, and to what extent. They can even predict where the rainforest will recede in the near future. The trick is in how the algorithms read the images.
Satellites and drones supply countless images of rainforests – from various altitudes, and in differing resolution and quality. What they have in common is that the regions they depict are not labelled or otherwise identified. Unlike maps, the places don’t bear names, and the forests, rivers and roads have no readily identifiable “signatures”, or “labels”, as a computer scientist would say. This means that computer algorithms are unable to discern what is forest coverage and what isn’t.
“Fish bones” show where forests are shrinking
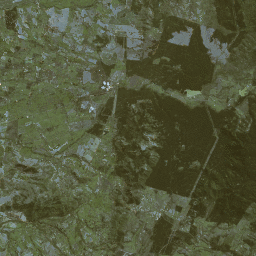
As Dao explains, the algorithms read sequences in order to recognise which areas are forested and whether these areas are shrinking. These sequences are individual images strung together in chronological succession – much like old film reels or comic strips. So when a new road is built through the rainforest, for instance, numerous smaller roads form off it over time. It is along these roads that the forest coverage is destroyed.
From a bird’s-eye view, the resulting pattern resembles the skeleton of a fish, with its spine and small bones – thus the moniker “fish bones”. By comparing these chronologically sequential aerial views, algorithms can determine how road systems and forest coverage change over time.
This means that intelligent algorithms don’t need labels to generate an overall image indicating where rainforests are shrinking. They can also predict where the worst deforestation will appear next. This model also applies to deforestation near rivers and around agricultural areas.
Test run in the Chilean rainforest
For the research project, which is called Komorebi, David Dao has attracted partners from the field, including Chile’s forestry authority CONAF (Corporación Nacional Forestal). In January, a pilot project will start in the Valdivian rainforest, on the Pacific coast south of the capital, Santiago. Dao will be testing and tweaking his predictive algorithms in real rainforest conditions. His approach may be able to detect not just overall decline in the rainforest, but also determine which species of trees are most affected.
This is a significant factor in climate change, as different types of trees store CO2 at different rates, and one approach to forest conservation is to offer local populations financial incentives for retaining trees as CO2storage rather than clearing the forest.
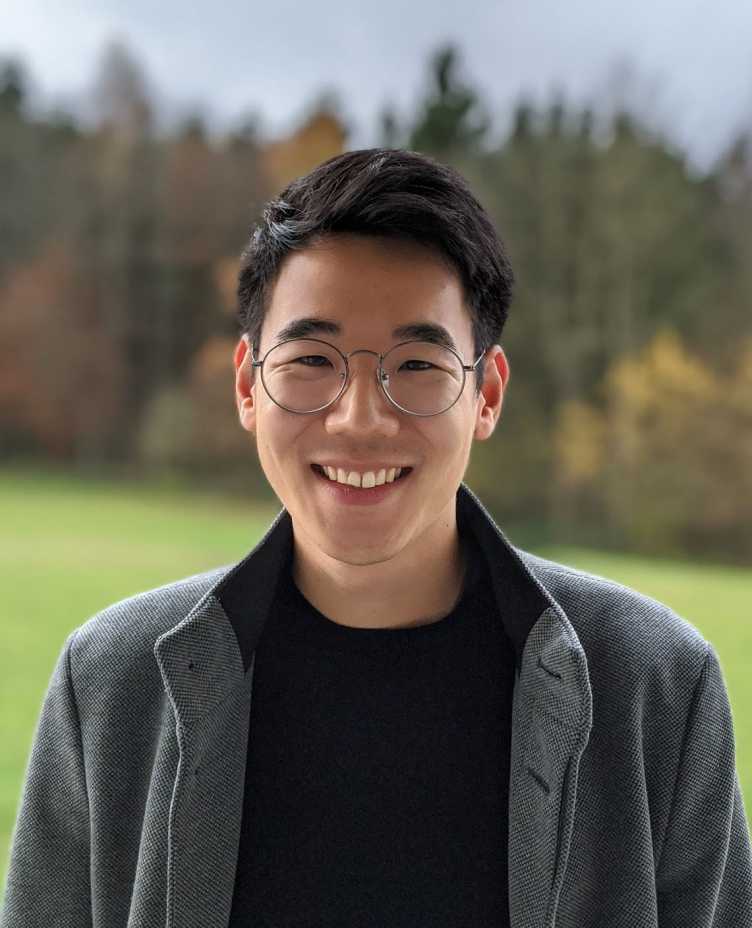
In the Chilean rainforest, they will examine issues such as how to improve the accuracy of predictive algorithms by combining satellite imagery with pictures captured by drones lower down. Unlike satellite imagery, drone images can be accurate to within 30 centimetres. “If we have drone images, we can also observe changes in tree species and detect changes in biodiversity,” says Dao. Today, David Dao will be presenting his research project at the 25th UN Climate Change Conference in Madrid (COP25).
The session, hosted by the Inter-American Development Bank and the Chilean forestry authority, will look at ways of using new technologies to record and predict changes in land use, as well as the potential to link the results to payments that local inhabitants receive for preserving the rainforest.
AI and climate financing at COP25
Alongside David Dao, other researchers from ETH Zurich are presenting their work in the areas of climate financing and machine learning at events during the UN Climate Change Conference 2019 (external pageCOP25call_made). They include Lynn Kaack, a postdoctoral researcher in the Energy Politics Group, who organised a panel discussion with the international research group “external pageClimate Change AIcall_made” in the Chilean pavilion. The panel discussed how artificial intelligence methods can be deployed for climate protection and the challenges this presents to research, industry and the public sector.
Lucas Bretschger, ETH Professor of Economics/Resource Economics, will lead the official Switzerland side event – with the Swiss delegation and his doctoral students Anna Stuenzi and Julia Bingler. The topic is climate finance, in particular the goal of aligning global financial flows with internationally agreed climate targets. This creates particular challenges for financial centres such as Switzerland, and for the regions most affected by climate change.