Improving quality through artificial intelligence
ETH researchers are using artificial intelligence to improve quality management in digital production processes. The team has succeeded in halving the proportion of defective products in an experiment with the semiconductor manufacturer Hitachi Energy.
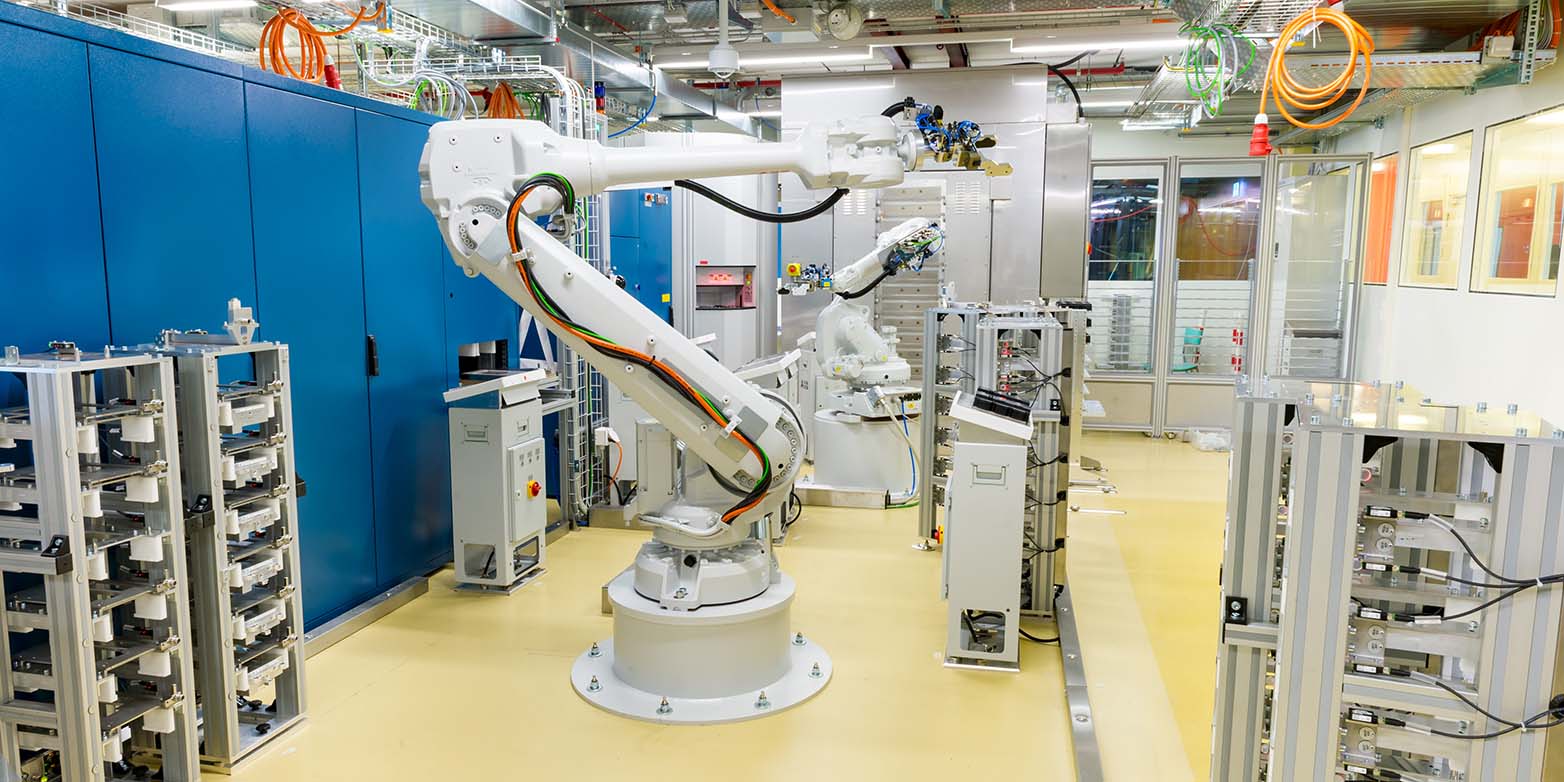
Complex machinery, pharmaceutical products or microchips: the more expensive a product, the more important its quality when it comes to surviving on the market. This is especially true for industrial production in high-wage countries like Switzerland. It is all the more surprising, then, that poor quality continues to account for an average of 15 percent of operating costs in industrial manufacturing, often as a result of outdated quality management.
In a new study, ETH researchers Julian Senoner and Torbjørn Netland together with Stefan Feuerriegel, who recently joined LMU Munich, highlight how artificial intelligence (AI) can be used to improve quality management in complex digital production processes. Their algorithm has already been successfully tested at a semiconductor factory of Hitachi Energy – previously Hitachi ABB Power Grids – in Lenzburg: the number of defective products was reduced by over 50 percent in one experiment, making production not only more efficient but also more sustainable.
Outdated quality management
The manufacture of complex industrial products requires a large number of interrelated work steps. Producing semiconductors, for example, for use in computers, trains or wind turbines, involves between 200 and 400 production steps. Various things can go wrong in any one of these steps. If the temperature in one machine is a little too high, or the pressure in another a little too low, for example, a large proportion of the products may be affected by errors. “In semiconductor production, it’s not uncommon for the error rate to range between 5 and 70 percent because of the highly complicated manufacturing process. There are huge amounts of money at stake,” explains ETH professor Torbjørn Netland from the Chair of Production and Operations Management.
Until now, it has been extremely difficult to precisely identify sources of error in complex production processes of this kind. Traditional methods used in quality assurance at many factories around the world only ever enabled engineers to examine a few parameters at a time. In complex production processes, however, thousands of interrelated parameters are now measured, but traditional methods did not allow for analysis of how these interact and affect overall production. ETH professor Netland and his co-authors are aiming to change this: “We want to bring quality management into the digital age. The methods that continue to be used by many producers are often up to 100 years old,” says Netland.
Algorithm identifies sources of error
First, the ETH researchers developed an algorithm that imitates the individual steps involved in the production of semiconductors. They then fed the algorithm with as much historical production data as possible, such as the temperature or pressure measured in machines. “Based on this data, the algorithm learns the conditions needed to ensure good semiconductor quality, and which conditions result in high error rates,” says Julian Senoner, lead author and a researcher in Netland’s group.
The advantage of the AI-based method is that it can be used to analyse any number of factors and relationships in the production process, and reveal more complex interrelationships between parameters. This enables sources of error to be identified in a more systematic way, and throughout the entire production process. The proposed approach will not obviate the need for well-trained engineers at the factories, though – on the contrary: “Our algorithm primarily identifies sources of error that have previously gone undetected. But fixing them will still take a great deal of technical expertise and human creativity,” Netland explains.
50 percent less loss
To test the algorithm, the authors worked with Hitachi Energy in Lenzburg. Hitachi Energy produces semiconductors that are used in electric vehicles and trains, as well as in wind turbines and power lines. Historical production data from the semiconductor manufacturer enabled the researchers to identify two manufacturing steps that most impaired the quality of the products.
Armed with these findings, they went to the factory to conduct an experiment under real production conditions. Together with Hitachi Energy engineers, the authors divided a production batch of 1,488 semiconductors into four groups of equal size. While the first 372 semiconductors were produced without specific adjustments, one manufacturing step each was optimised for groups two and three, and both manufacturing steps were then optimised for the last 372 semiconductors. “The results of the experiment confirmed the predictions made by the algorithm: in the control group produced without optimisation, the error rate was four times higher than in the group produced according to the algorithm’s recommendation,” says ETH professor Netland.
Hitachi Energy subsequently implemented the ETH researchers’ recommendations in another product variant, and reduced the proportion of defective products by more than half. For the semiconductor manufacturer from Lenzburg, the algorithm developed by the ETH researchers offers significant benefits that will help to improve quality management in a meaningful and lasting way.
Transferable to other sectors
But are these results also feasible in other industries? “A lot of production data is needed for our method to achieve good results, and this calls for highly digitalised production processes,” explains ETH researcher Senoner. According to the authors, these conditions are currently found above all in the pharmaceutical and chemical industries and in plastic production.
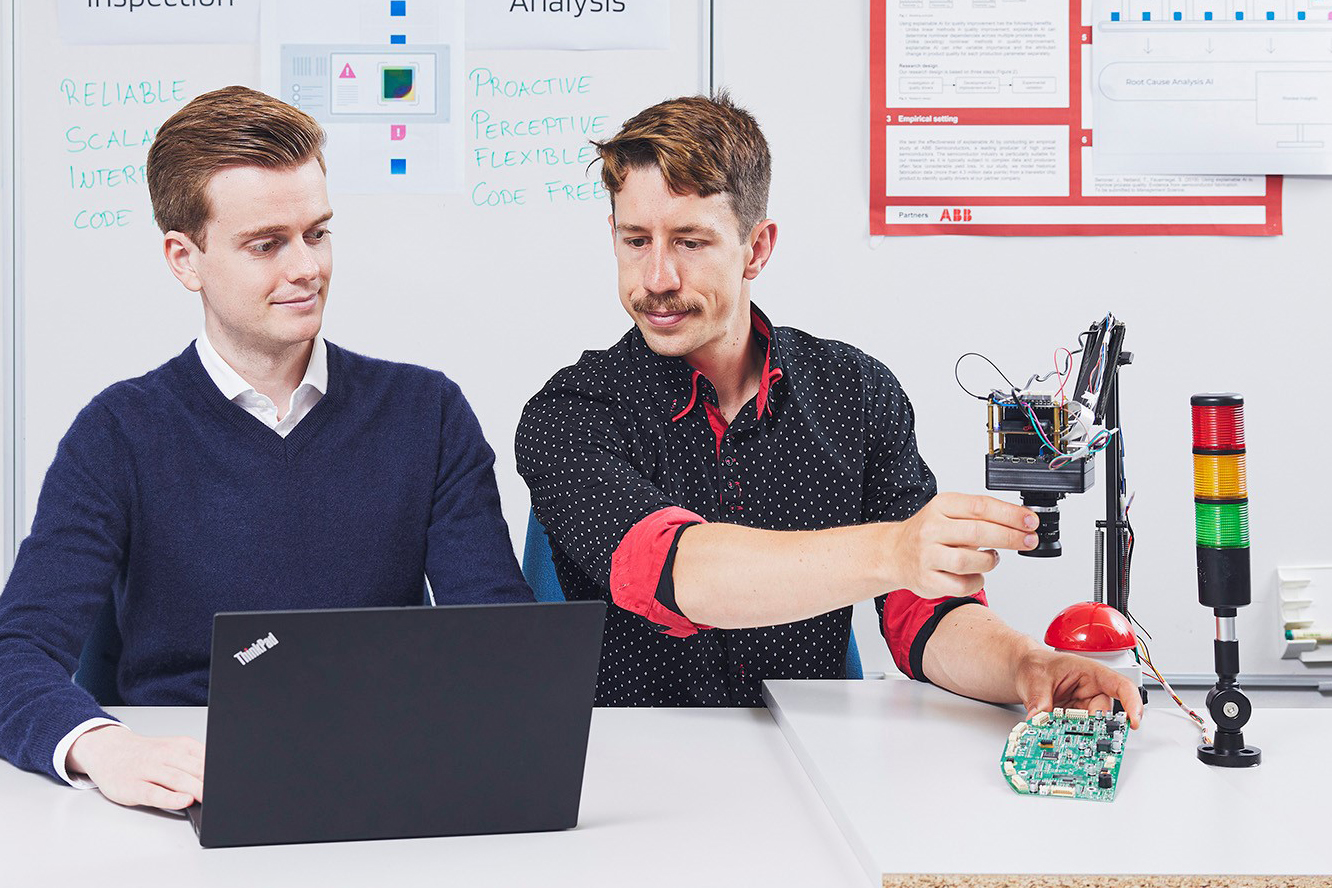
With the increasing digitalisation of production processes, however, the ETH researchers’ algorithm should also become attractive for other sectors in the medium term. With a view to exploiting the economic potential of artificial intelligence in quality management and making the technology accessible as widely as possible, lead author Julian Senoner and ETH Pioneer Fellow Bernhard Kratzwald have therefore founded the ETH spin-off external pageEthonAIcall_made.
Reference
Senoner J, Netland T, Feuerriegel S (2021). Using explainable artificial intelligence to improve process quality: Evidence from semiconductor manufacturing. Management Science, published online: 9 December 2021. DOI: external page10.1287/mnsc.2021.4190call_made